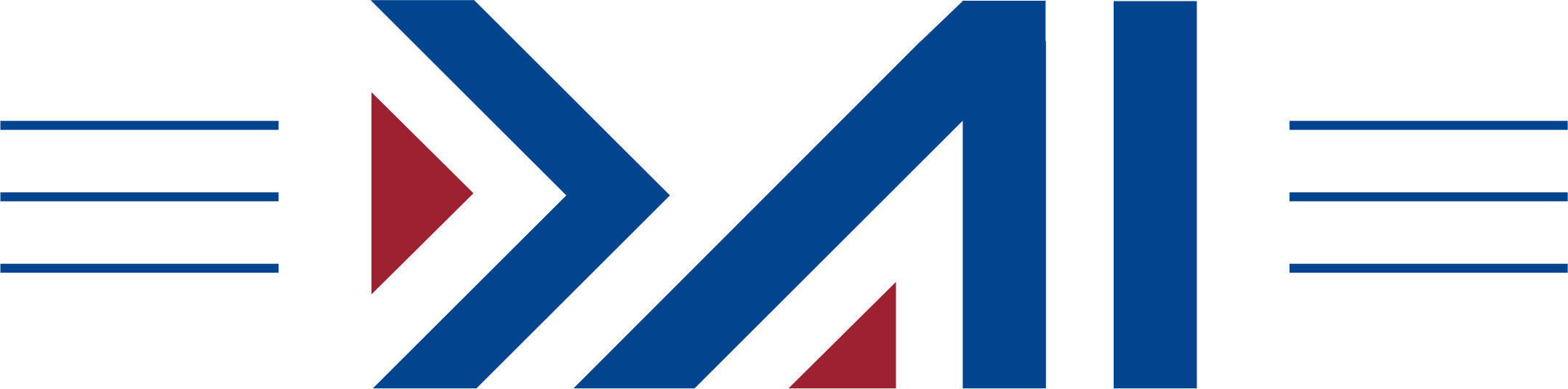
|
Prof.
SHEN's Group
Distributed
Artificial Intelligence Laboratory, ERC-FCDE, MoE
School of Mathematics, Renmin
University of China
|
Home | Research
| Honors
|
Teaching
|
Publications
| Members
| Future Students
| ILC DataBase
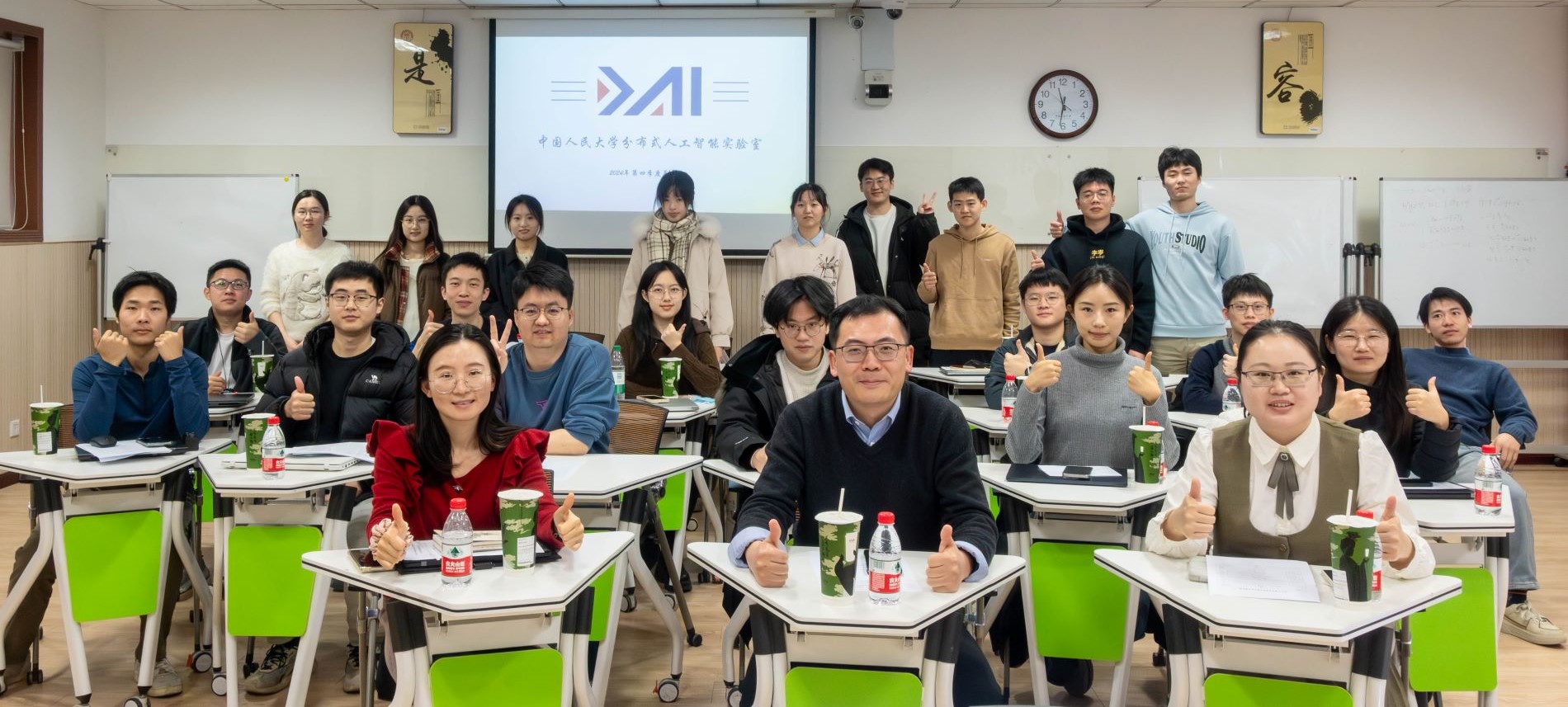
● About us
● News
[20250709]
The paper titled "Fractional-proportional-type Iterative Learning
Control With a Novel Gain Selection Rule" has been accepted for
publication in IEEE Transactions on Cybernetics.
[20250619]
The paper titled "Input fluctuation mitigation in coupled nonlinear
control systems: Adual-mode DMPC approach" has been accepted for
publication in Systems & Control Letters.
[20250618]
The paper titled "scRECL: Representative Ensembles with Contrastive
Learning for scRNA-seq data clustering analysis" has been accepted for
publication in Briefings in Bioinformatics.
[20250609]
The paper titled "Finite-Iteration Learning Control for Nonlinear
Systems with Parameter Uncertainties" has been accepted for publication
in IEEE Transactions on Systems, Man, and Cybernetics: Systems.
[20250601]
The paper titled "Noisy Error-adaptive Weighting Strategy for
Accelerating ILC in Discrete-time Systems" has been accepted for
publication in IEEE Transactions on Cybernetics.
[20250527] Congratulations to Shuai Gao for Qualifications for Government-Sponsored Study Abroad by the China Scholarship Council.
[20250527] Prof. Jinjun Shan visited our group upon invitation and delievered a leture "Adaptive Decision-making of Autonomous Driving Vehicles".
[20250505]
The paper titled "Dual-variable iterative learning control for switched
systems with iteration experience succession strategy" has been
accepted for publication in Automatica. This paper was led by Prof. Yiwen Qi and collaborated with Prof. Choon Ki Ahn.
[20250325] Prof. Shen was selected as one candidate of 2024 Most Cited Chinese Researchers by Elsevier.
[20250302]
The paper titled "Consensus control for multi-agent systems with
unknown faded neighborhood information via iterative learning scheme"
has been accepted for publication in Information Sciences.
[20250221] Prof. Abdelhamid Tayebi visited our group upon invitation and delievered a lecture "State Observers Design for Autonomous Navigation Systems".
[20250207]
The paper titled "Distributed Closed-loop Reference Adaptive Learning
Control For Parallel Mutual Inductance Circuits" has been accepted for
publication in IEEE Transactions on Circuits and Systems II: Express Briefs.
[20250207] Prof. Alessandro Astolfi
visited our group upon invitation and delivered a lecture "Pontryagin
meets Bellman: on combining Pontryagin's Principle and Dynamic
Programming".
[20250109] Prof. Shen was invited to join the Journal of the Franklin Institute editorial board as an Associate Editor.
[20250105] Congratulations! Dr candidates Zeyi Zhang and Zihan Li have been selected in Youth Talent Support Program for Doctoral Students launched by China Association for Science and Technology. They are supported by Chinese Mathematical Society and Chinese Association of Automation, respectively.
[20250101] The DAI Lab
Annual Report for 2024 has been released.
[20250101] We, DAI Lab,
wish you Happy 2025.
● Research
Highlights: Variable Gain Design in Learning Control
We
consider nonlinearity and randomness as two primary challenges in
addressing practical application problems, among other potential
challenges. Nonlinearity implies limited available information on
system dynamics, but this can be effectively addressed by ILC due to
its inherent data-driven control feature. Randomness is another fundamental characteristic in the practical
problems, as reflected by practical disturbances, measurement noise,
communication noise, and unknown uncertainties, which are often
represented by random variables and necessitate the application of
probability theory for proper handling. This poses a significant challenge in
stochastic ILC. To tackle this issue, variable gain design emerges as a
promising approach.
Decreasing Gain:
The decreasing gain indicates a variable gain sequence that gradually
diminishes to zero as the iteration number increases. The conditions
for the decreasing gain have been established to ensure asymptotic
convergence in the iteration domain, serving as the fundamental
cornerstone and support for subsequent results.
Adaptive Gain:
The adaptive gain aims to divide the learning process into two stages:
an initial stage employing a fixed gain and a later stage transition to
a randomly diminishing gain sequence. Here, the switching iteration
between these two stages is adaptively determined by the learning
process itself; furthermore, the diminishing gain is closely tied to
practical learning processes rather than being predefined.
Event-triggering Gain:
The event-triggering gain aims to construct a successive sequence of
learning stages, with reduction in learning gains occurring only when
transitioning from one stage to another. This perspective offers a
completely new view on the learning process.
Optimal Gain:
The optimal gain seeks an optimal value of the learning gain based on
specific performance indices related to the learning process. One
approach involves approximating mean-squared input error to generate
optimal gain values, while another employs Kalman filtering principles
to generate a recursive sequence of gain matrices.
For more details, please refer to Variable Gain Design in Stochastic Iterative Learning Control.
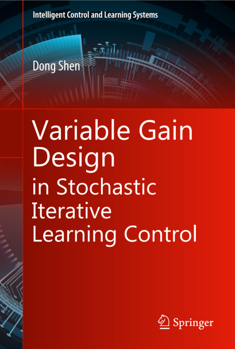
● Annual
Reports
Wu
Yuzhang Distinguished Professor Dong Shen
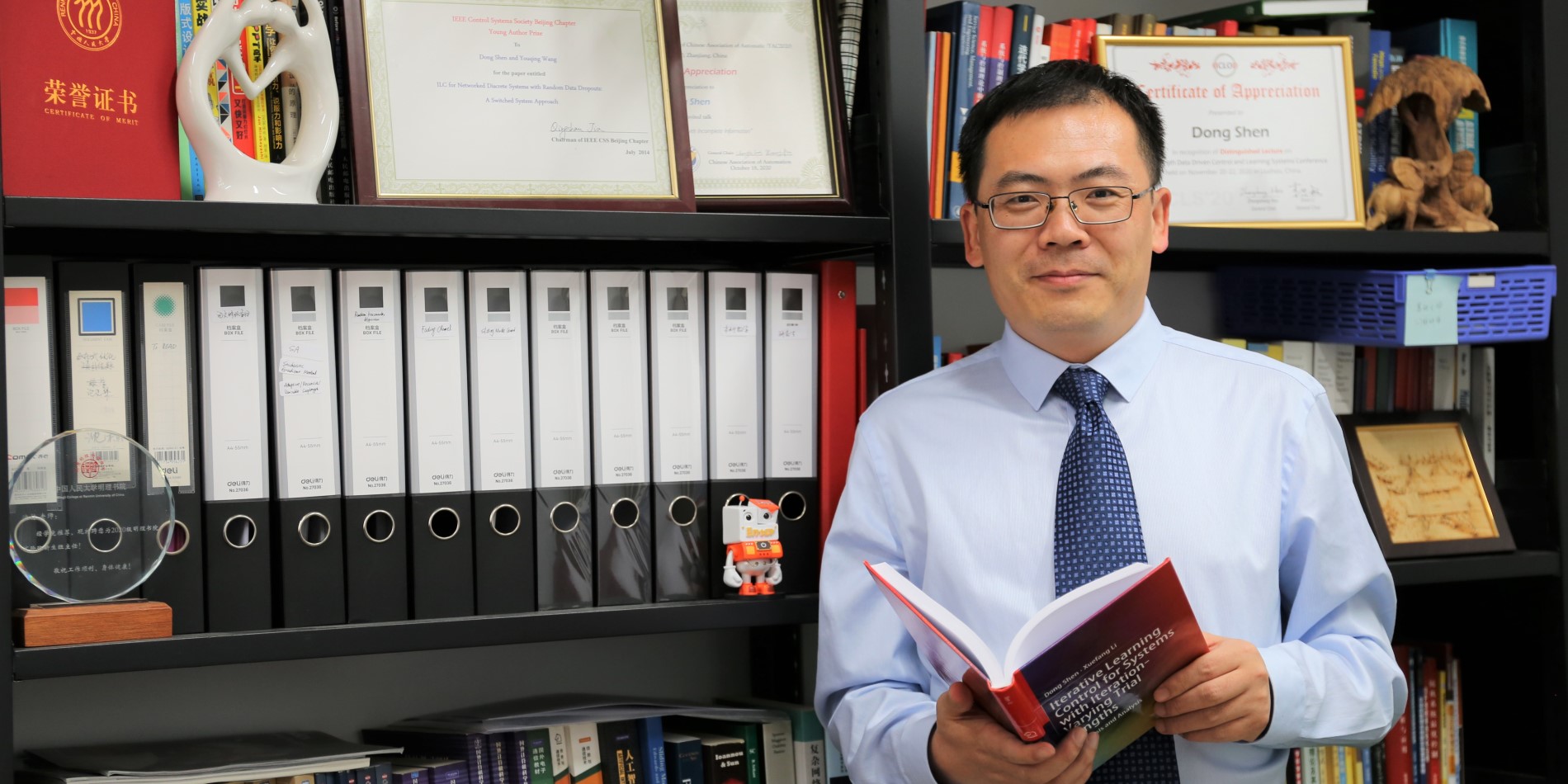
● Contact Information
Office
Address:
Room 207, No. 4
Teaching Building, Renmin University of China, No. 59
Zhongguancun Street, Haidian District, Beijing 100872
Mailing
Address:
School of
Mathematics, Renmin University of
China,
No. 59 Zhongguancun Street, Beijing 100872, P.R. China
Tel: 86-10-82507078
E-mail: dshen [at] ieee [dot] org
ORCID: 0000-0003-1063-1351
● Education
2005.09-2010.07,
Ph.D. in Mathematics, Academy of Mathematics and Systems Sciences,
Chinese Academy of Sciences
2001.09-2005.07, B.S. in Mathematics, School of Mathematics, Shandong
University
● Professional
Positions
2020.01-present,
Professor, School of Mathematics, Renmin University of China
2020.01-present, Professor, Eng. Res. Center of Finance
Computation and Digital Engineering, Ministry of Education
2020.01-present, Principal Investigator, Distributed Artificial Intelligence Lab.,
ERC-FCDE, MoE
2019.07-2019.08, Visiting Scholar, RMIT University, Australia
2012.06-2019.12,
Associate Professor, Beijing University of Chemical Technology
2016.02-2017.02, Visiting Scholar, National University of Singapore,
Singapore
2010.07-2012.05, Postdoctoral Fellow, Institute of Automation, CAS
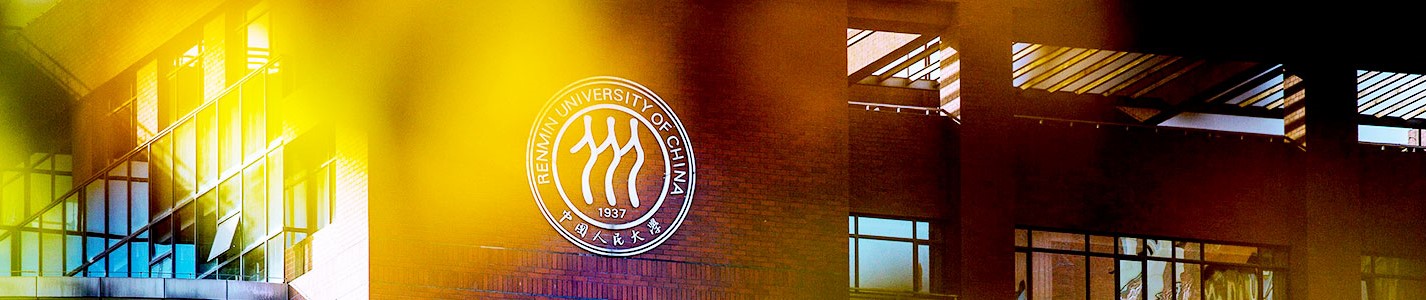